Improving machine learning with a multiscale Gaussian process
Applying a novel approach to the Gaussian process is yielding more accurate prediction models and strengthening machine-learning capabilities.
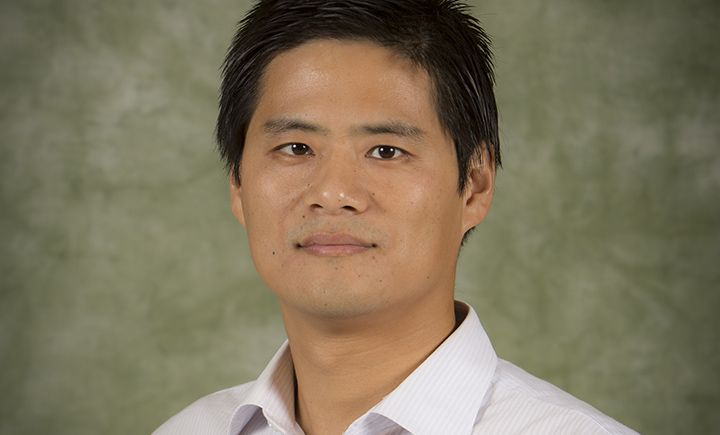
In November 2017, Assistant Professor Changqing Cheng of the Systems Science and Industrial Engineering Department won the Best Paper Award in Data Mining and Decision Analytics at the INFORMS Annual Meeting.
His paper, titled “Multiscale Gaussian process for dynamic evolution prediction of complex systems,” takes a step toward improving machine learning for data that is nonlinear and nonstationary by using a nonparametric multiscale Gaussian process model.
“The widespread use of smart devices [has] enabled the acquisition of a vast array of data and dramatically lowered the cost of information storage and retrieval,” explains Cheng. “This has boosted the application of analytics including research in machine learning and pattern recognition in various engineering and business domains.”
With an ever-growing collection of data, machines are able to learn and predict patterns more accurately and clearly. However, there are still some systems that are difficult to analyze because of things like the complexity of the relationships or the variety of contributing factors.
“For most real-world complex systems, it is extremely difficult to specify the relationship between input and output,” Cheng says. “In such cases, nonparametric models [like the Gaussian process] are helpful toward aiding modeling selection.”
Cheng’s paper introduces a novel way to use the Gaussian process for understanding complex systems.
His research imposes the Gaussian process model “on each proper rotation and baseline generated from ITD [intrinsic time-scale decomposition].”
In the paper, Cheng applies his multiscale model approach to two case studies, one using synthetic and the other using real-world data.
For both cases, Cheng’s multiscale Gaussian process model outperforms the conventional alternatives by at least 20 percent.
Cheng says that his model is “particularly effective for multistep forecasting of nonlinear and nonstationary time series.”
Having a model that can more accurately predict complex systems means that machine-learning overall improves, and the possibilities of what can be converted into a predictive network expand.