Binghamton researchers blend different AI systems for better autonomous driving
Watson College team combines task, motion planning to improve vehicle performance
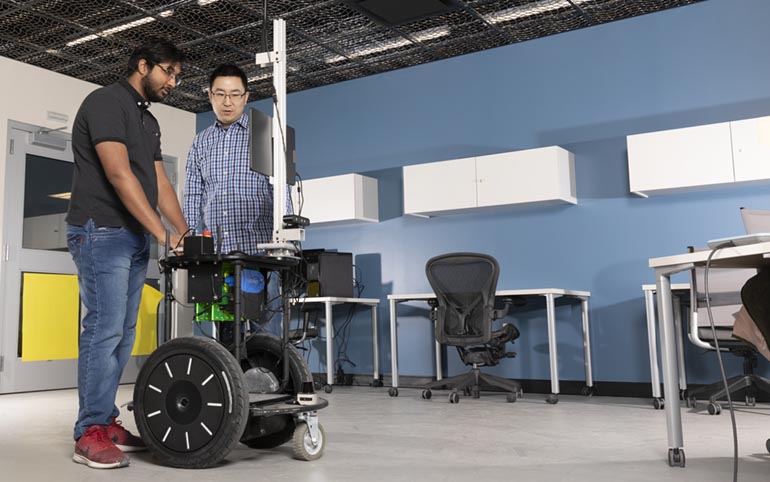
Vehicles that drive themselves have been a trope of science fiction films and television shows for decades, but technology is finally catching up to those long-held aspirations.
Researchers continue to explore the best ways for artificial intelligence to navigate busy streets without causing traffic accidents — and a new paper from Binghamton University combines two ideas to better meet that goal.
Assistant Professor Shiqi Zhang — a faculty member in the Thomas J. Watson College of Engineering and Applied Science’s Department of Computer Science — guided a trio of his students for a paper titled “Task-Motion Planning for Safe and Efficient Urban Driving.”
The paper’s first author, Yan Ding, is a second-year PhD student; the second author, Xiaohan Zhang, earned a master’s degree in computer science at Watson in the spring and started the PhD program this fall; and the third, Xingyue Zhan, is an undergraduate.
The Watson team proposes bringing together two AI models, Zhang said: “This research, for the first time, enables active communication between the task level that determines where to go to accomplish the whole task, and the motion level that computes trajectories to drive the vehicle from one point to another.”
To evaluate different scenarios, a typical AI runs many different options in parallel and decides to try one of them in the real world. Based on how that goes, it will give each simulation a different score. For instance, it may try using a robot arm to pick up a cup without knowing how much the cup weighs, and then reconsider once it discovers that information.
By marrying task planning to motion planning, many more possible solutions can open up. One scenario that Zhang uses to explain this new research is telling an autonomous vehicle to go to a certain retailer. If traffic is bad or the store is busy, the AI can suggest a similar retailer further down the road. It evaluates situations from two distinct pathways to offer the best outcome.
“A lot of research has been focused on the safety of autonomous driving, but one thing that’s frequently overlooked is the fundamental tradeoff between safety and efficiency,” he said. “We can always take a longer route and be super-safe, but it would take two hours to get home.”
To test their theories, the Watson team used CARLA, a program independently created and maintained by the automotive industry that simulates various autonomous-vehicle situations, such as traffic patterns, pedestrian behaviors, weather conditions and sensor data.
“My students spent a week running the research in the simulator, trying many different scenarios,” Zhang said. “We finally got a few failure cases. Even in the real world, we drive for many years but hopefully only have accidents once or twice. In the simulation, we can catch these situations and revise the programming to avoid the accidents in the future.”
The CARLA simulator allows researchers to try all kinds of “crazy behaviors” without any real-world consequences. Even experiments in Zhang’s robotics lab are run with care, with one or two students always ready to hit a “stop” button to prevent damage to the office environment or the robots themselves.
Zhang and his students are using the conclusions from this new study to explore further avenues of research, which could include real-world tests for their AI software.
“It’s probably too much to have a real autonomous vehicle and drive it on the road in Binghamton, but we’re thinking of building an in-house autonomous driving environment and using our small robots to try simulations in the real world,” Zhang said.
“The challenge is that multi-robot scenarios are not easy. You can’t just control this one — you have to play the role for the surrounding vehicles. That’s the next thing I want to try, but I probably need some support from the University to give us an indoor space for such experiments.”
Zhang’s lab also is collaborating with Ford Motor Co.‘s autonomous driving team on improving rider experience, and it received two Ford University Research Program awards in 2019 and 2020.