Computer science professor wins NSF grant to improve real-time data services
Professor KD Kang received $599,084 for research to improve smart transportation, healthcare, manufacturing and other key functions
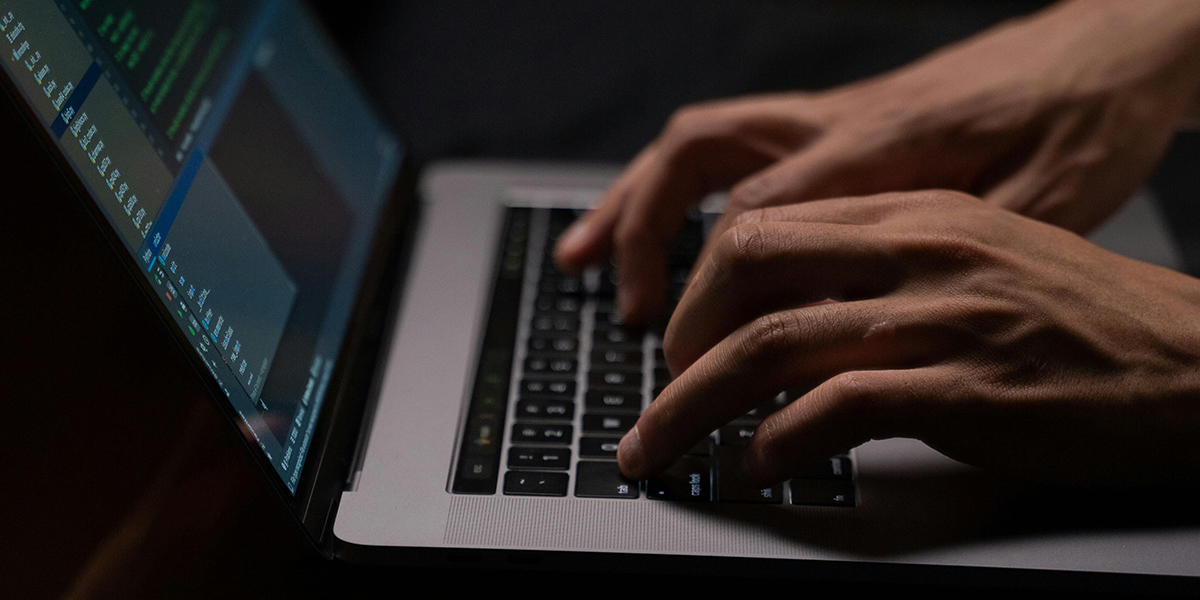
Having real-time data at our fingertips has become such an expected thing in the internet age that most of us don’t think about how it happens — and how to make it better.
That’s where computer science researchers like Professor KD Kang can help.
Kang — a faculty member at Binghamton University’s Thomas J. Watson College of Engineering and Applied Science — recently received a $599,084 grant from the National Science Foundation to improve real-time data services that support smart transportation, healthcare, manufacturing and other key functions.
To be most useful to users, real-time data services need to fulfill three important mandates: The data must be as fresh as possible, it needs to be processed in an efficient way, and the system should not consume too much energy.
“My research is trying to support these three requirements, but sometimes they conflict with each other,” Kang said. “For example, if you want to maximize freshness, you can update the data very frequently, but that takes more resources. Other tasks or queries that need to be processed in a timely manner may miss deadlines, and it also increases power consumption. I want to strike a balance between those three conflicting requirements, and that could have important applications in the real world.”
Calling it an “underexplored area of research,” Kang will leverage advanced memory hardware features and real-time data characteristics to enhance timeliness and reduce processor and memory power consumption.
For instance, he wants to use machine learning to better prioritize what data is most needed and how it is processed, as well as aggregating tasks with similar data needs and consolidating them to one CPU core. That allows idle cores to be turned off to reduce power consumption.
Much of the work for the NSF grant will be trying different combinations of freshness, processing speed and power requirements to find the right balance among all three that provides optimum performance.
“Near the end, I will have a prototype system that can target a specific application such as vehicle sensors — something that has a nice set of sensors and a lot of real-time data analysis requirements, as well as tight power-consumption requirements,” Kang said. “Compared to current systems, I can hopefully enhance timeliness and freshness but reduce power consumption by, say, 20%. That’s my goal. Then we can take what we learn and apply it in other contexts.”